Big data can be applied in the supply chain, but do you know how? Keep reading and discover the 3 applications of Big Data in supply chain management (SCM).
Big Data
Broadly speaking, Big Data refers to large volumes of data, both structured and unstructured. It can be analyzed for insights and applied in machine learning (ML) projects, predictive modeling, and other advanced analytical applications. Big Data analysis uncovers hidden patterns, unique correlations, and critical insights that wouldn’t be reachable with traditional data analysis.
Examples of Big Data include data found in business transaction systems, customer databases, medical records, Internet click logs, mobile applications, and social networks.
But what is the difference between traditional data and Big Data?
Traditional data is structured data in fixed formats or fields in a file stored in a centralized database architecture. This data is relatively easy to maintain in all types of companies, from start-ups to large associations. Common database tools such as SQL (Structured Query Language) are used to access this data.
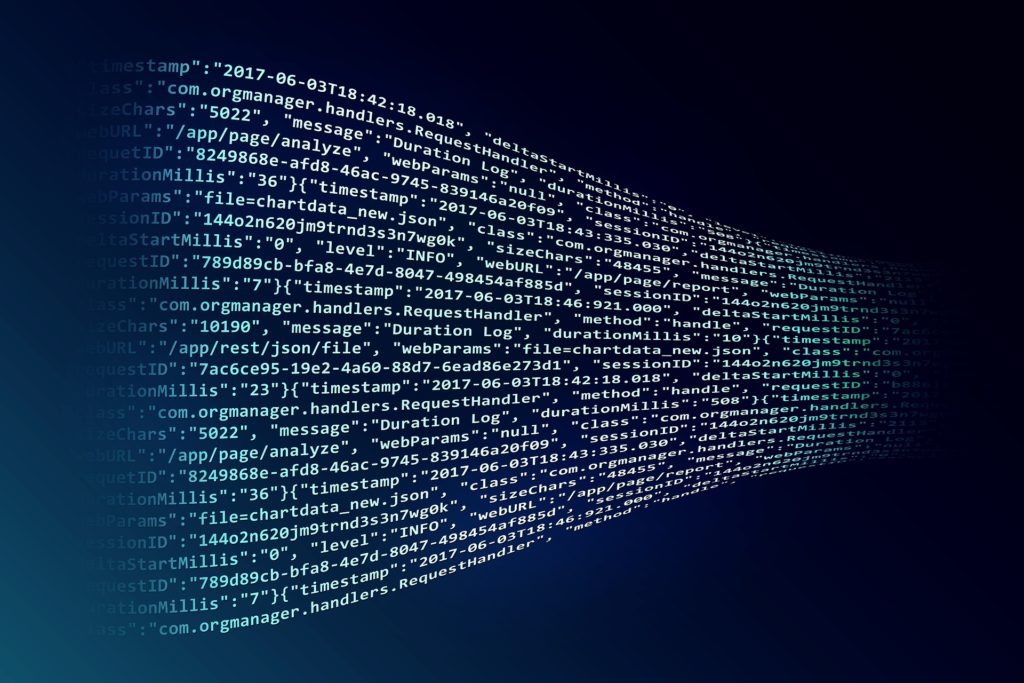
Big Data in the supply chain: why is it important?
In the context of international logistics, interest in Big Data is resurrecting. In a research published by Production, 60% of interviewers claimed that they use Big Data applications to supply chain management.
However, does your supply chain need Big Data? It expands the info set for analysis beyond the traditional internal data stored in corporate resource planning and supply chain management systems. For instance, throughout the supply chain, point-of-sale info, inventory data, production volume data, weather conditions, and social data. Also, other unconventional data points can be analyzed to suggest end-to-end supply chain improvements.
Therefore the 3 applications of Big Data in the Supply Chain that the article suggests are:
1) Improving operations and reducing costs
From suppliers to manufacturers, including distributors, carriers, freight forwarders, retailers, and consumers; all these participants in the logistics process interact with each other generating large data sets. These ones can be used for optimization projects. Therefore, Big Data analytics is able to improve. : Demand forecasts, reduce safety stocks, generate optimal delivery plans and reduce the cost of uncertainty.
For example, Big Data analytics help minimize delivery delays by analyzing GPS data. As well as weather and traffic data, to optimize delivery routes. United Parcel Service (UPS) uses an internal dynamic route optimization system that has helped it reduce the number of wasted miles on delivery routes, all thanks to the value of Big Data advances.
2) Strategic business development
Supply chain Big Data can be applied to planning and decision-making in the boardroom. A great example of this application is when Big Data analytics has been a valuable tool for manufacturing companies to develop strategies, share data, design predictive models, and plan factory networks. It has also proven to be crucial for the design and development of new products and innovations.
3) Improved customer experience
When Big Data is effectively used, it can dramatically increase customer satisfaction by enabling companies to identify their customers’ preferences or pain points. Besides, it can be valuable data that is difficult to obtain directly from the consumer.
As a result of this application, companies are able to analyze social media, mobile, and web data to learn how customers use their products. In more innovative cases, other companies have explored the use of unified systems to monitor on-shelf stock levels.
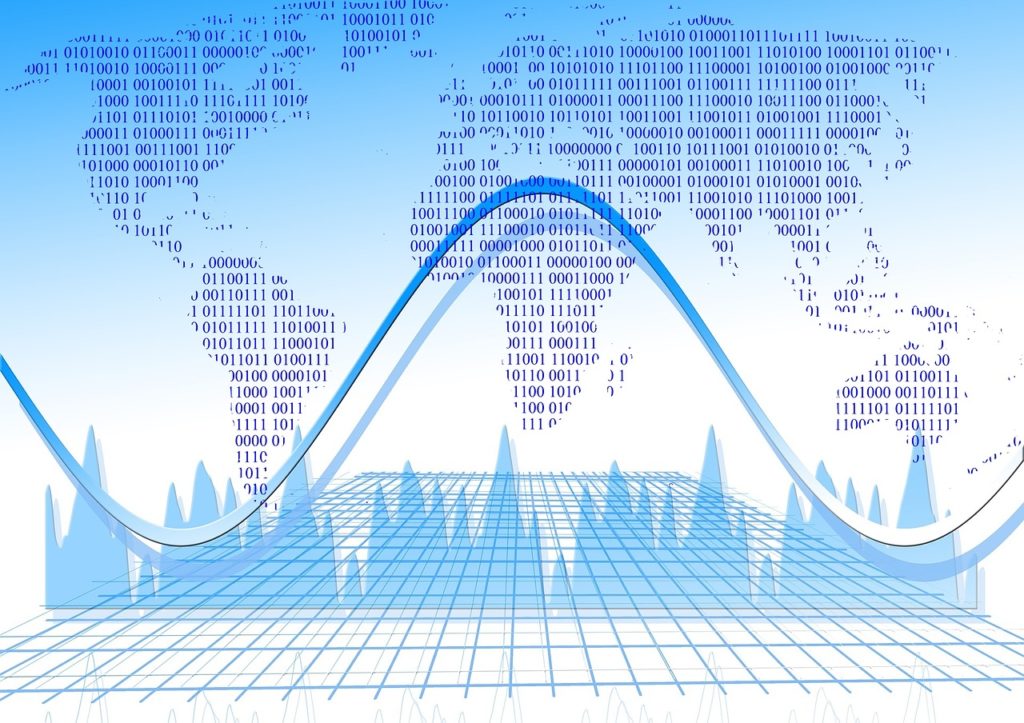
Big Data has arrived to stay
Big data can be the key to creating profitable, customer-centric supply chains. Indeed, the importance of big data cannot be overstated; despite its notoriety as another industry buzzword, its impact is here to stay.